

Ultimately, prediction accuracy and reliability are greatly improved compared to algorithms’ performances without the framework’s operation. Results indicated that through data preprocessing and resampling, Machine Learning algorithms performance is enhanced. Various aspects during this process will be thoroughly examined such as the bias-variance tradeoff and the problem of overfitting, data transforms, feature engineering and selection, ensemble methods as well as pursuing optimal resampling towards better model accuracy. The target is to build a framework that will preprocess input data, compare the results among a group of Machine Learning algorithms and propose the best-predicted value of economic profit. Real data from the farms and the weather in the area will be used. The case study focuses on olive tree farms located on the Hellenic Island of Crete. The objective is to estimate the optimal economic gain while preserving water resources on irrigation by considering various related factors such as climatic conditions, crop management practices, soil characteristics, and crop yield. This paper presents an end-to-end Machine Learning framework for predicting the potential profit from olive farms. In combination with the climatic change, those are major obstacles to maintaining sustainable development, especially in a semi-arid land.

Consequently, water resources consumed on irrigation are increased. The research results show a great potential application for a future intelligent trading platform which provides traders with real-time data driven market analysis and accurate predictions on market trends and commodity trading prices.Īgricultural systems are constantly stressed due to higher demands for products. Based on our comparative evaluation results, the proposed model outperforms other existing models. As a result, our proposed agriculture trading models for crops (corn, oats, soybean, and soybean oil) based the improved LSTM provide great accurate prediction results (>0.95%).
Real time commodity risk engine machine learning series#
The paper presents three improved machine learning models based on LSTM, GRU, and SARIMA, and proposes an advanced deep learning LSTM model with time series and multi-variant features to forecast crop market trends with predicted prices. This paper focuses on this demand by studying and comparing existing machine learning models for intelligent trading and price prediction. However, current trading platforms lack AI-powered solutions to support traders and investors with dynamic commodity market analysis based on historical big data, accurate forecasts on market trends and trading price forecasts. To stabilize food price and better understand and predict the market trend, people need a real-time efficient online platform as an intelligent trading tool to support dynamic agriculture commodity trading.
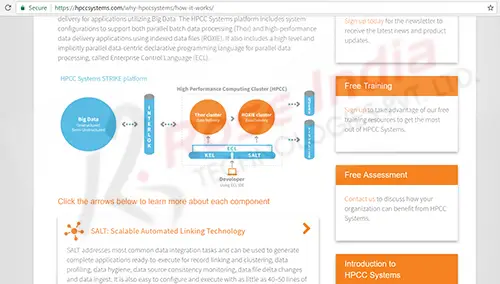
This suggests that food, agriculture, and global commodity trading are essential and critical to human beings. The global population is expected to reach 9.7 billion in 2050, according to the UN News. Particularly in the long-term experiment testing, the average performance of LSTM with MSE has improved 45.5% while ARIMA has dropped 74.1% and the average MSE of LSTM is 0.304 which outperformed all other four algorithms. The results validate the efficiency of the proposed Long Short-Term Memory Model (LSTM) to serve as the prediction engine for the proposed system. In the two series experiments, five popular machine learning algorithms, ARIMA, SVR, Prophet, XGBoost and LSTM have been compared with large historical datasets in Malaysia.
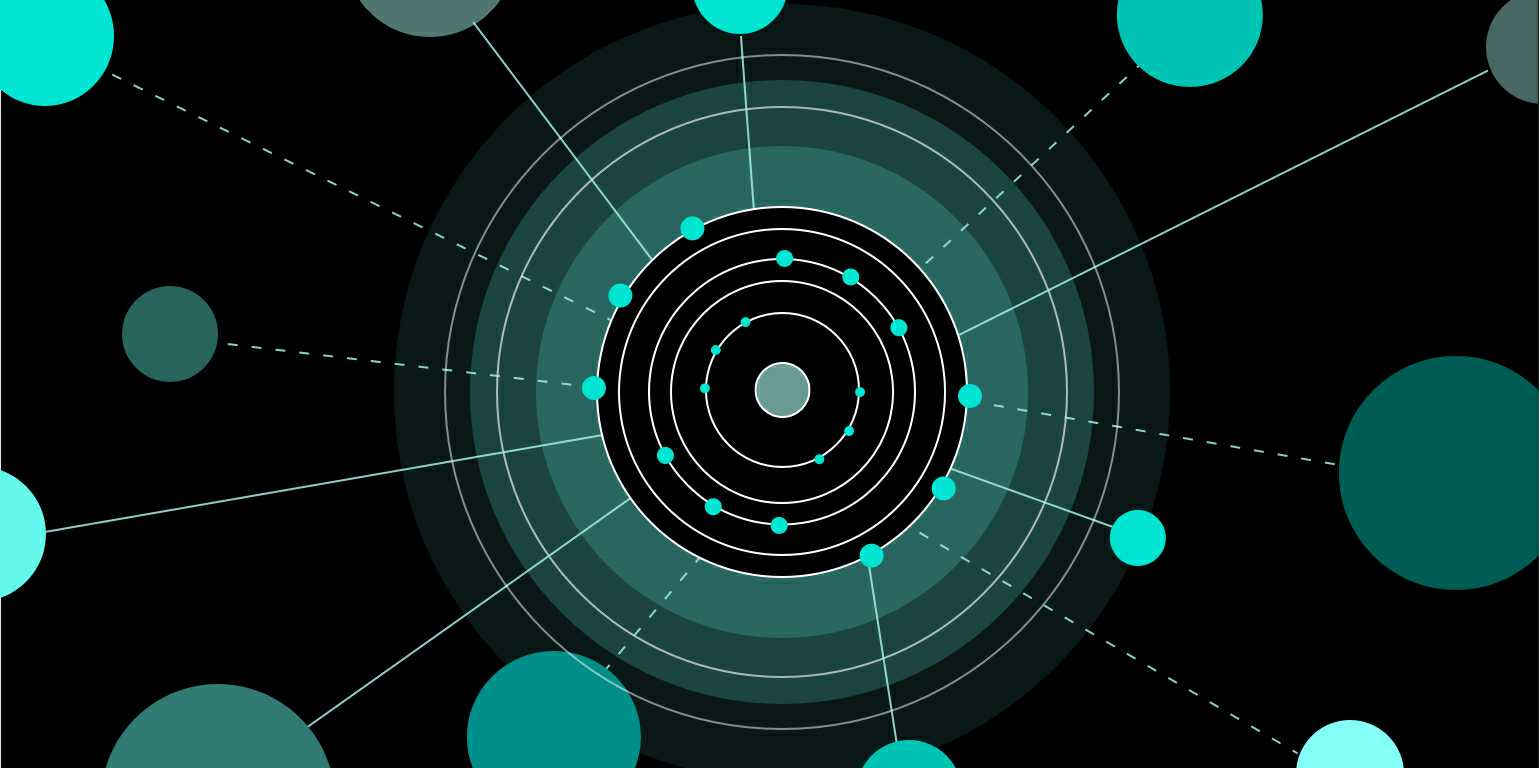
In this research, we address these problems by conducting a machine learning strategy study and propose a web-based automated system to predict agriculture commodity price. On the other hand, when implementing machine learning techniques, finding a suitable model with optimal parameters for global solution, nonlinearity and avoiding curse of dimensionality are still biggest challenges. However, the selection of proper machine learning techniques for automated agriculture commodity price prediction still has limited consideration. Due to the increasing large amounts historical data of agricultural commodity prices and the need of performing accurate prediction of price fluctuations, the solution has largely shifted from statistical methods to machine learning area. The intention of this research is to study and design an automated agriculture commodity price prediction system with novel machine learning techniques.
